The power of real-world qualitative assessments for addressing climate security risks
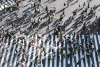
Qualitative research can uncover hidden drivers and connectors, reveal unintended consequences, and generate contextualised and actionable knowledge by delving into the lived experiences, perceptions, values, and behaviours of people and communities most affected by climate change.
The Weathering Risk (WR) methodology combines quantitative and qualitative research in a people centred, intersectional and gender-disaggregated approach, meaning it recognises and integrates different groups' unique experiences and perspectives. Using state-of-the-art spatially disaggregated climate impact data and analysis in combination with qualitative methods conducted by local teams on the ground such as ethnographic fieldwork, participatory mapping, focus groups, focused community consultations, multi-stakeholder dialogues and conflict sensitivity assessments, we gain a more nuanced understanding of climate security issues and can develop actions to address them. The WR methodology focuses on context and actor-specific entry points for tailoring solutions to the needs of communities and policymakers swiftly.
Quantitative advancements in the climate-conflict nexus research
Climate scientists, economists, geographers, among others, have created complex models that rely on big data to explain the climate-conflict nexus. Agronomists use geospatial data to have effective crop production and yield optimisation methods. Countless efforts have been made to understand climate change's adverse effects on the risk of violent conflict, combining rainfall variability and satellite imagery with machine learning (ML). Satellite data can provide insights into deforestation patterns in areas that are difficult to access due to conflict. This data facilitates hot-spot mapping in regions most exposed to the adverse effects of climate change and can indicate any overlap of climatic stress and socio-political fragility. In general, big-data techniques offer an increased capacity to explore relationships between a large number of variables that may contribute to violent conflict. Nevertheless, different factors hinder empirical research with such sophisticated models.
Data quality and subtle information
There are many constraints regarding the quality of data used to feed ML models that show trends on climate-related security risks. Although in recent years, climate data has improved in its quality and availability, there are still challenges in finding granular data on socio-political changes and conflict. The contextual dynamics in state-society relations, which mediate extreme weather events and violence, are determined by factors like culture, religion, identity, income inequality and the adaptability of institutions. These factors and nuances should be investigated through qualitative techniques to promote rapid action even if an algorithm has not been perfected. In-depth qualitative methods can uncover the nuances in local contexts.
As an illustration, available data on violence and the variables used by ML that define and eventually even determine the existence of violent conflict have different gaps in the datasets used. First, the robustness and precision of the data vary depending on the reporting source. Authoritarian governments often do not report human rights violations, which compromises the legitimacy of the datasets and their comparability. Second, slow-onset social unrest is more challenging to represent through proxy variables that show the progression of conflict. For example, ACLED is a comprehensive database, which has been one of the most frequently used for climate-conflict research, uses the number of reported violent deaths in the dataset. Nevertheless, data like this does not necessarily allow to capture the full complexity of common climate-security pathways.
Insights from the Weathering Risk initiative have shown climate change affects agricultural production and food price volatility, possibly evolving social unrest, which often manifests in (violent) protest. Suppose the only conflict-related variables considered as a sign of social unrest come from direct violence, such as violent deaths during protests. In that case, the model's predictability will diminish the seriousness of any social movement without clear evidence of violent protest. For example, the involvement of women as informants in conflict dynamics, which is not necessarily present in quantitative data can – and should – be integrated into analysis using qualitative methods. Similarly, low-level community conflicts often interact with drivers of recruitment or support to armed groups. However, these interactions can take many different shapes. The dynamics of recruitment at the beginning of a cascade of incidents that involve low-key participation in radical groups do not necessarily show evident signs of violence early on.
There have been developments to overcome the challenge of integrating climate-conflict dynamics in models. Research using data on radical political attitudes under environmental stress points such as droughts has been conducted. The findings show that fragile state-citizen relations are essential in linking drought exposure and support for political violence, especially evident in politically marginalized communities. Similarly, a growing number of similar publications using algorithms to model the stages of conflict/protest escalation has shown light on social unrest events sequencing. Such techniques have not been used extensively in climate and conflict research, which could be a new research avenue.
Qualitative knowledge, co-creation methodologies and qualitative assessments help uncover the hidden intentions and behaviours of stakeholders. The face-to-face interaction and human empathy both allow for a more profound understanding of the local context, even in the case of non-verbal communication. Furthermore, these types of interactions typically reduce prejudice among parties, enabling them to have a neutral space for dialogue to frankly look for solutions and search entry points where conflicting parties may see themselves collaborating towards climate and conflict resilient pathways. Nevertheless, qualitative methodologies might also be biased. For instance, when respondents answer based on what they think is the socially desirable thing to say in the context, or when differences in power relationships occur within a focus group or consultation.
Using a climate justice perspective to enhance capacities and share data to transform conflicts
Additionally, there are also more practical obstacles that make relying on quantitative data problematic. Machine learning models require considerable investments and adequations on the ground to collect and standardise data. Such efforts are more challenging to implement in fragile contexts. Conflict-affected countries and the most fragile societies typically lack funds, developed monitoring systems and data infrastructure. Additionally, much of the highly local economic activity happens in unrecorded informal markets.
A climate justice perspective suggests to invest in the local capacities to build and use climate-conflict nexus models and coupled big-data findings with local-qualitative assessments to foster resilience. A complementary strategy can be to integrate local communities in data collection and co-creation where different methodological approaches can foster synergistic and transformative solutions that consolidate local-based analysis, capacities, ownership and resources to respond accordingly. Communities have a wealth of information and knowledge on climate change impacts on farming systems and endogenous climate-resilient crops; however, this information must be collected systematically and translated into diverse knowledge approaches. Promoting data collection and sharing and community monitoring empowers local communities and serves them as advocacy tools to participate in tailoring better responses to needs. These can have a significant impact if communities, in coordination with national early warning systems and policies, use the information to tailor planning processes and actionable strategies. Even more, there are clear indications that participatory community processes of climate monitoring can open safe spaces for dialogue between conflicting parties if undertaken with a conflict-sensitive lens.
From another perspective, in conflict-affected contexts, issues related to emotional trauma and different types of victimization play a role in how communities react to a changing climate and build their responses to various challenges. Adelphi’s experience, working on climate security in Pacific Island states, shows how communities actually experiencing sea level rise often disregard new climate-predictive models and instead, demand more action-oriented responses to cope with the issue. Similarly, the emotional processes involved in post-conflict peacebuilding are not easily quantifiable. The complexities of national reconciliation and transitional justice processes, or how addressing environmental and climate change-related challenges enable avenues of trust and collaboration, must be understood and supported by real people and a deep understanding of local values.
Human behaviour is not easily predictable
Although behaviour patterns and suggestions about the probability of conflict risk can be inferred from quantitative data, numerous psychological (internal), sociological, and environmental factors influence human nature and wellbeing. Not even the most advanced models with the most considerable volume of data can predict human will and erratic reasoning under imminent threats that are posed by climate change. A critical moderating variable between climate-related disasters and violent conflict is governance and the history of conflict. The political dynamics in a conflict-affected country show complex relationships where political negotiations, political economy, cultural features, national identities and communal perceptions of different social groups play an immense role. These factors cannot be measured and quantified easily. Therefore, qualitative methodologies that embrace the power of local knowledge should complement big-data efforts.
Understanding past conflict dynamics to prevent new conflicts
To ensure sustainable transitions, it is important to take preventive actions and engage in multi-stakeholder dialogue that involves private, public, and civil society groups. This approach should be qualitative, localised, and inclusive. In most cases, previous conflict dynamics can affect the emergence of new conflicts, which further interact with politics surrounding climate change mitigation, adaptation, and disaster risk reduction. For example, there is sufficient evidence about the disputes that emerge over land and water around mining. With the projected increase in demand for critical materials needed for low-carbon energy production, such conflicts may become even more prevalent without proper safeguards and dialogue processes in place. Qualitative assessments can help facilitate such processes and prevent these conflicts from emerging. It is particularly true in Latin America and Africa, regions where climate changes have already affected water security and mining operations and which have an abundance of critical materials required for the energy transition.
The qualitative power of local data and responses
Weathering Risk relies on the potential of finding solutions at the local level with a global perspective. It requires acknowledging the agency and expertise of local populations, who, under harsh conditions and with many structural vulnerabilities, still come forward with innovative ideas to address the complex challenges of compounding crises. These local actions should be recognised through qualitative research that understands the challenges faced by local communities and their perspectives on the climate and conflict-related issues affecting them.
It is not enough to recognise trends and risks to prevent conflict effectively. We need to allocate more resources to locally driven action. Qualitative assessments should be conducted even before a data model for predicting climate-related conflict is perfected. Stakeholders involved in conflict prevention and climate action can benefit from understanding the governance and institutional adaptability of early warning systems and anticipatory action. This is especially important for climate adaptation efforts, which should be integrated into the daily activities of vulnerable communities and sub-national politics. We must understand local power and social dynamics and capacities to bring about real change. Communities are essential sources of knowledge, innovation, and impactful climate adaptation, and they are the driving force behind a transformative, resilient agenda for sustainable transformation.